4 Ways Retrieval Augmented Generation (RAG)
is
Making a Splash
Retrieval-Augmented Generation (RAG) is a cutting-edge approach to generating natural language. It combines retrieval methods with generative models to create high-quality, informative text. While RAG is impressive, it’s not a one-size-fits-all solution. This article explores four areas where RAG truly shines: factual accuracy, reducing hallucinations, domain-specific applications, and offering transparency.
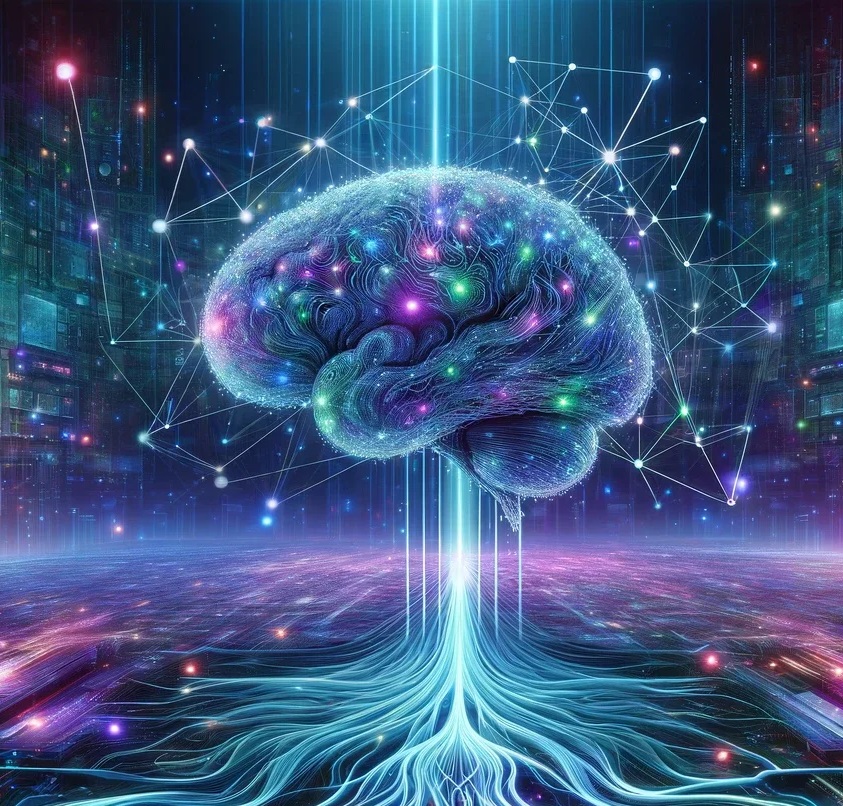
Demanding Accuracy
As AI becomes more integrated into various fields, concerns like compliance and liability arise. Here, RAG excels at generating factually correct and contextually consistent text. This makes it ideal for tasks like:
- News Summarization: Keeping up with current events can be overwhelming. AI can summarize news at a high level, but inaccurate information can lead to poor decisions. RAG ensures summaries are factually accurate.
- Scientific Reporting: Researchers are embracing AI for generating scientific reports. RAG allows researchers to focus on other aspects while producing concise, accurate summaries for colleagues.
- Medical Information Summarization: For patients with complex medical histories, RAG helps physicians navigate patient flow with up-to-date information.
- Legal Document Summarization: Similar to scientific reports, legal documents are often lengthy and complex. RAG can condense legal documents referencing vast amounts of laws and precedents into understandable summaries.
- Financial Reporting: The financial world is data-heavy. RAG applications can sift through massive datasets to produce concise, accurate reports in a fraction of the time it takes a human.
Combating Hallucinations
A common problem with traditional NLG models is “hallucination,” where the model generates text not supported by the input data. RAG mitigates hallucinations by grounding the generation process in real-world evidence. It retrieves relevant documents from a large corpus to inform generation, significantly reducing the likelihood of hallucinations.
Tailored for Specific Domains
RAG can be fine-tuned to specific domains, allowing it to generate text tailored to those domains’ requirements. This is particularly valuable for industries looking to leverage AI specific to their field. RAG allows models like ChatGPT, Llama, and Gemini to specialize in a particular domain, improving text quality and reducing hallucinations for domain-specific topics.
Transparency and Explainability
Unlike many NLG models, RAG is relatively transparent and explainable. You can understand how the model generates text and identify the sources of its information. This allows for easier debugging and ensures the model produces accurate and reliable text.
Conclusion
RAG is a powerful and versatile NLG technique with a wide range of applications. Its ability to ensure factual accuracy, mitigate hallucinations, be transparent, and specialize in domains makes it a valuable tool for many tasks.