Imagine an AI writer not merely weaving words from its own internal loom, but meticulously selecting threads from the vibrant tapestry of human knowledge. This is the realm of retrieval-augmented generation (RAG), a revolutionary technique transforming how language models process information and create content.
Traditionally, large language models (LLMs) have served as isolated libraries, drawing solely on their training data for text generation. This approach often resulted in factual inconsistencies, biased pronouncements, and the occasional foray into fabricated information.
RAG introduces a game-changer: external knowledge retrieval. The LLM becomes a curious scholar, venturing into vast data repositories like Wikipedia, scientific publications, or even news articles. A dedicated research assistant (replacing “retriever”) scours these resources, unearthing relevant documents that enrich the LLM’s understanding of the prompt.
Think of it this way: an LLM tasked with writing a blog post on climate change might once have offered generic facts. With RAG, the research assistant retrieves articles on the latest IPCC report, specific impacts on ecosystems, and personal stories from individuals affected. Armed with this diverse and current information, the LLM generates a nuanced, factual, and potentially impactful piece.
But the benefits of RAG extend beyond mere accuracy. It unlocks doors to:
Enhanced trust and transparency: Knowing the sources behind the LLM’s output fosters confidence. Each fact can be traced back to its source, verified for authenticity, and the model can be held accountable for its claims.
Unleashing creativity with grounded imagination: RAG doesn’t stifle creativity; it fuels it. The LLM, inspired by real-world data, can conjure more grounded, relevant, and even surprisingly creative content. Imagine fiction based on actual historical events, poems echoing real-world struggles, or code generated with specific applications in mind.
Democratizing knowledge access: With RAG, LLMs can tap into vast knowledge repositories not limited to their training data. This empowers them to tackle problems and generate content in domains they may not have previously engaged with.
Of course, RAG faces its own challenges. Biased retrieved data can perpetuate bias in the model’s output. Efficiently filtering and selecting relevant information remains a hurdle. Ensuring the authenticity and trustworthiness of external sources is crucial.
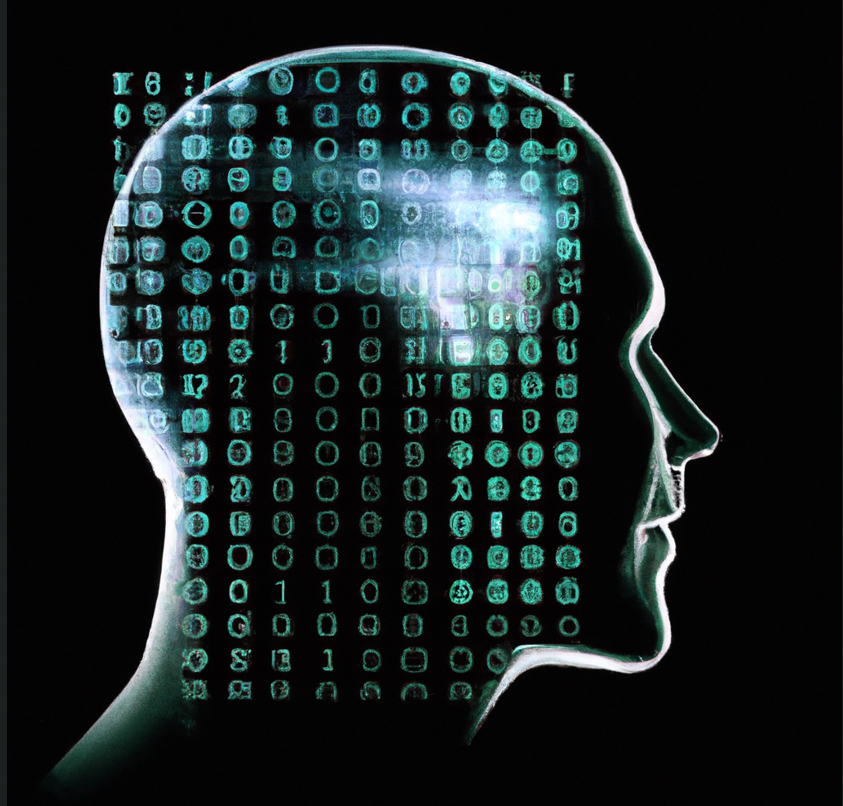
But these are challenges worth tackling. As we navigate the evolving landscape of AI, RAG offers a promising path towards LLMs that are not simply clever mimics, but informed collaborators in learning, creating, and understanding the world around us.
So, the next time you encounter an AI-generated piece that feels surprisingly insightful or well-informed, remember: it could be the work of an AI scholar, diligently crafting its words from the rich tapestry of human knowledge. And that’s undoubtedly something to celebrate.
Now, it’s your turn! What excites you most about retrieval-augmented generation? What challenges do you foresee? Share your thoughts in the comments below!
Imagine an AI writer not merely weaving words from its own internal loom, but meticulously selecting threads from the vibrant tapestry of human knowledge. This is the realm of retrieval-augmented generation (RAG), a revolutionary technique transforming how language models process information and create content.
Traditionally, large language models (LLMs) have served as isolated libraries, drawing solely on their training data for text generation. This approach often resulted in factual inconsistencies, biased pronouncements, and the occasional foray into fabricated information.
RAG introduces a game-changer: external knowledge retrieval. The LLM becomes a curious scholar, venturing into vast data repositories like Wikipedia, scientific publications, or even news articles. A dedicated research assistant (replacing “retriever”) scours these resources, unearthing relevant documents that enrich the LLM’s understanding of the prompt.
Think of it this way: an LLM tasked with writing a blog post on climate change might once have offered generic facts. With RAG, the research assistant retrieves articles on the latest IPCC report, specific impacts on ecosystems, and personal stories from individuals affected. Armed with this diverse and current information, the LLM generates a nuanced, factual, and potentially impactful piece.
But the benefits of RAG extend beyond mere accuracy. It unlocks doors to:
Enhanced trust and transparency: Knowing the sources behind the LLM’s output fosters confidence. Each fact can be traced back to its source, verified for authenticity, and the model can be held accountable for its claims.
Unleashing creativity with grounded imagination: RAG doesn’t stifle creativity; it fuels it. The LLM, inspired by real-world data, can conjure more grounded, relevant, and even surprisingly creative content. Imagine fiction based on actual historical events, poems echoing real-world struggles, or code generated with specific applications in mind.
Democratizing knowledge access: With RAG, LLMs can tap into vast knowledge repositories not limited to their training data. This empowers them to tackle problems and generate content in domains they may not have previously engaged with.
Of course, RAG faces its own challenges. Biased retrieved data can perpetuate bias in the model’s output. Efficiently filtering and selecting relevant information remains a hurdle. Ensuring the authenticity and trustworthiness of external sources is crucial.
But these are challenges worth tackling. As we navigate the evolving landscape of AI, RAG offers a promising path towards LLMs that are not simply clever mimics, but informed collaborators in learning, creating, and understanding the world around us.
So, the next time you encounter an AI-generated piece that feels surprisingly insightful or well-informed, remember: it could be the work of an AI scholar, diligently crafting its words from the rich tapestry of human knowledge. And that’s undoubtedly something to celebrate.
Now, it’s your turn! What excites you most about retrieval-augmented generation? What challenges do you foresee? Share your thoughts in the comments below!